How can AI optimize system integration
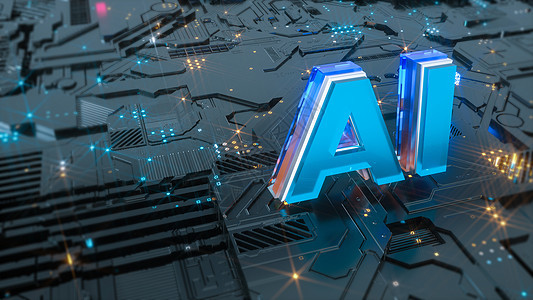
While the speed of the manufacturing process has reportedly slowed, the complexity of chip design has not decreased. For chip designers, in such a process, if AI cannot be used for itself in the era of drive, it will undoubtedly stagger the efficiency of itself and even the entire design project. Although everyone has noticed this, so AI is starting to infiltrate EDA's tools at this stage, what design challenges can AI solve?
Verify
Verification is one of the biggest challenges in chip design. We have seen a trend towards expensive special verification hardware and clouds, which is enough to show that verification is a resource-consuming process in chip design. This refers not only to hardware computing resources, but also to time resources. Validation may take even longer than other processes. In recent years, the number of verification engineers on many chip development teams has also gradually increased, growing even faster than design engineers. However, few in the industry have optimized the verification process.
AI This gap finally loosened, many manufacturers began to use AI to optimize the verification process, such as through coverage prediction and incentive optimization to accelerate coverage standards. Cadence also released the VerisiumAI drive verification platform this year, according to Cadence, the emergence of Verisium means that SoC verification has changed from a single run single engine algorithm to a single run single engine algorithm AI multi-run multi-engine algorithm to assist big data, reduce debugging turnaround time and improve debugging efficiency. Automatic classification of failed test cases reduces manual analysis effort.
Analog design automation
Compared with digital ics in terms of design, analog IC design is obviously much worse in terms of automation. In the design process of digital circuit, the degree of freedom of the whole process is gradually reduced. Although the same is true of analog circuit design, the decline is no better than that of digital circuit design, especially in terms of layout and verification, so automation is not yet on the agenda. At present, the design of analog circuits largely depends on the designer's own intuition, skill and experience.
With the help of AI, the EDA tool provides advanced machine learning algorithms under the training of large amounts of data, so that the layout and routing of analog circuits have a more efficient automated process, especially in constraint extraction and generation, layout optimization, optimization of analog circuits, generation and simulation verification of AI acceleration. In this way, the number of iterations per analog circuit design will be further reduced, and the time-to-market of the chip will also be shortened.
System integration
In recent years, the popularity of UCIe, Chiplet, 3D packaging, etc., is actually a system integration concept. The introduction of design and manufacturing optimization schemes, such as DTCO and so on, has also attracted more and more attention. How to integrate more transistors, more memory and logic + memory integration and ultimately the thermal management of software-combined hardware are all issues that need to be considered in system integration.
So how can AI optimize system integration? The answer is to provide a more efficient route to explore the design space, which can give predictive models and faster implementation methods, such as New Thinking DSO.ai and FusionComplier, which provide complete AI for better design solutions, design solutions PPA and faster design acceptance. It is known that these solutions from Syrs have recently been validated in TSMC with N3E for high-performance computing, where AI compute-intensive loads, such as mobile devices, provide enhanced power consumption, performance and yield.
Peroration
In fact, once chip design enters the AI-assisted era, design engineers are also putting forward correspondingly higher requirements, because many low-level design problems have been solved by AI prediction, optimization, and generation. Design engineers need to innovate at higher levels of design, such as joint optimization of systems/software, so that they are not denied "optimization" falls. In the future, the professional skills of design engineers will be more inclined to the skills that data scientists need to master, and data scientists may have the opportunity to seize the work of design engineers.
Die Produkte, an denen Sie interessiert sein könnten
![]() |
FLTR100V10 | LINE FILTER 75VDC 10A TH | 217 More on Order |
![]() |
MPR0854FPXXXZ01A | AC/DC CONVERTER 54V 800W | 5868 More on Order |
![]() |
AXH010A0Y93-SR | DC DC CONVERTER 1.8V 18W | 7272 More on Order |
![]() |
AXH010A0MZ | DC DC CONVERTER 1.5V 15W | 6318 More on Order |
![]() |
ATS020A0X3-SRPHZ | DC DC CONVERTER 0.8-3.63V 73W | 8676 More on Order |
![]() |
SC003A0A91-SR | DC DC CONVERTER 5V 15W | 5274 More on Order |
![]() |
SW003A0A91-SRZ | DC DC CONVERTER 5V 15W | 7578 More on Order |
![]() |
QW050B71 | DC DC CONVERTER 12V 50W | 7650 More on Order |
![]() |
QRW035A0F1 | DC DC CONVERTER 3.3V 116W | 8928 More on Order |
![]() |
QHW075A71 | DC DC CONVERTER 5V 75W | 7092 More on Order |
![]() |
MH010BK | DC DC CONVERTER +/-12V 10W | 4770 More on Order |
![]() |
JRW040A0A1 | DC DC CONVERTER 5V 200W | 5220 More on Order |
![]() |
JC100A | DC DC CONVERTER 5V 100W | 8298 More on Order |
![]() |
AXH016A0X3-SR | DC DC CONVERTER 0.8-3.6V 58W | 3456 More on Order |
![]() |
ATH016A0X43-SR | DC DC CONVERTER 0.8-3.6V 58W | 4698 More on Order |
![]() |
ESTW010A0B41-HZ | DC DC CONVERTER 12V 120W | 5004 More on Order |
![]() |
EHHD020A0F641Z | DC DC CONVERTER 3.3V 66W | 6084 More on Order |
![]() |
UDT020A0X43-SRZ | DC DC CONVERTER 0.45-5.5V | 4500 More on Order |
![]() |
SSTW001A3B41-SRZ | DC DC CONVERTER 12V 15W | 4626 More on Order |
![]() |
SSTW003A0A41-SRZ | DC DC CONVERTER 5V 15W | 8100 More on Order |
![]() |
APTS006A0X4-SRZ | DC DC CONVERTER 0.6-5.5V 33W | 8730 More on Order |
![]() |
SSTW005A0F41-SRZ | DC DC CONVERTER 3.3V 17W | 6732 More on Order |
![]() |
QBVW033A0B41-HZ | DC DC CONVERTER 12V 400W | 38 More on Order |
![]() |
APTS012A0X3-SRZ | DC DC CONVERTER 0.7-5.5V 66W | 451 More on Order |